基于小波包分解和卷积神经网络的滚动轴承故障诊断
Fault diagnosis of rolling bearing based on wavelet packet decomposition and convolutional neural network
-
摘要: 针对旋转机械滚动轴承在恶劣工作环境中易于损坏,而目前故障诊断过于依赖人工特征提取的问题,提出了一种基于小波包分解和卷积神经网络(CNN)的滚动轴承故障诊断方法(WPDEC-CNN):通过小波包分解对振动时域信号进行处理,获得表征信号相似的小波系数,再将其进行预处理后输入CNN进行分类识别.试验结果表明,WPDEC-CNN的损失率低于BP神经网络和CNN,为0.108 9;WPDEC-CNN的故障分类准确率均高于BP神经网络和CNN,达到97.3%,验证了所提故障诊断方法的有效性.Abstract: Aiming at the problems that the rolling bearing of rotating machinery is liable to be damaged in bad working environment,and the fault diagnosis at present relies too much on manual feature extraction,a rolling bearing fault diagnosis method based on wavelet packet decomposition and convolutional neural network (WPDEC-CNN) was proposed.This method used wavelet packet decomposition to process the vibration time-domain signal to obtain wavelet coefficients that characterized the signal similarly, and then preprocessed them and input them into CNN for classification and recognition.The experimental results showed that the loss rate of WPDEC-CNN was 0.108 9, which was lower than that of the BP neural network and the CNN.The fault classification accuracy of WPDEC-CNN reached 97.3%, higher than that of the BP neural network and the CNN, which verified the effectiveness of the proposed fault diagnosis method.
-
-
[1]
付阶辉.基于Petri网的故障诊断方法研究[D].南京:东南大学,2004.
-
[2]
张润林.旋转机械故障机理与诊断技术[M].北京:机械工业出版社,2002.
-
[3]
李舜酩,郭海东,李殿荣.振动信号处理方法综述[J].仪器仪表学报,2013,34(8):1907.
-
[4]
WANG J,MA Y,ZHANG L,et al.Deep learning for smart manufacturing:methods and applications[J].Journal of Manufaturing Systems,2018(48):144.
-
[5]
MATEO C,TALAVERA J.Short-time Fourier transform with the window size fixed in the frequency domain[J]. Digital Signal Processing,2018(77):13.
-
[6]
YANG Z J,YANG L H,QING C M.An oblique-extrema-based approach for empirical mode decomposition[J].Digital Signal Processing,2010(20):699.
-
[7]
ZHANG K,GENCAY R,YAZGAN M.Application of wavelet decomposition in time-series forecasting[J].Economics Letters,2017(158):41.
-
[8]
VAUTRIN D,ARTUSI X,LUCAS M,et al.A novel criterion of wavelet packet best basis selection for signal classification with application to brain-computer interfaces[J].IEEE Transactions on Biomedical Engineering,2009,56(11):2734.
-
[9]
QIN Y.A new family of model-based impulsive wavelets and their sparse representation for rolling bearing fault diagnosis[J].IEEE Transactions on Industrial Electronics,2018,65(3):2716.
-
[10]
DING X,HE Q.Energy-fluctuated multiscale feature learning with deep ConvNet for intelligent spindle bearing fault diagnosis[J].IEEE Transactions on Instrumentation and Measurement,2017,66(8):1926.
-
[11]
张建宇,李文斌,张随征,等.多小波自适应阈值降噪在故障诊断中的应用[J].北京工业大学学报,2013,39(2):166.
-
[12]
赵元喜,胥永刚,高立新,等.基于谐波小波包和BP神经网络的滚动轴承声发射故障模式识别技术[J].振动与冲击,2010,29(10):162.
-
[13]
JIA F,LEI Y,LIN J,et al.Deep neural networks:a promising tool for fault characteristic mining and intelligent diagnosis of rotating machinery with massive data[J].Mechanical Systems and Signal Processing,2016,72/73:303.
-
[14]
GAO R,YAN R.Wavelets[M].Boston:Springer,2011.
-
[15]
XUE J,XU S,SHUI P.Knowledge-based target detection in compound Gaussian clutter with inverse Gaussian texture[J].Digital Signal Processing,2019,95:102590.
-
[16]
TANG Z R,ZHU R H,LIN P,et al.A hardware friendly unsupervised memristive neural network with weight sharing mechanism[J].Neurocomputing,2019,332(7):193.
-
[17]
SMITH W,RANDALL R.Rolling element bearing diagnostics using the case western reserve university data:a benchmark study[J].Mechanical Systems and Signal Processing,2015,64/65:100.
-
[18]
LIU Y,YAO L,XIA Z,et al.Geographical discrimination and adulteration analysis for edible oils using two-dimensional correlation spectroscopy and convolutional neural networks (CNNs)[J].Spectrochimica Acta Part A:Molecular and Biomolecular Spectroscopy,2021,246(5):118973.
-
[19]
HUANG W Y,CHENG J S,YANG Y.Rolling bearing fault diagnosis and performance degradation assessment under variable operation conditions based on nuisance attribute projection[J].Mechanical Systems and Signal Processing,2019,114(1):165.
-
[20]
JIANG W,ZHOU J,LIU H,et al.A multi-step progressive fault diagnosis method for rolling element bearing based on energy entropy theory and hybrid ensemble auto-encoder[J].ISA Transactions,2019(87):235.
-
[1]
-
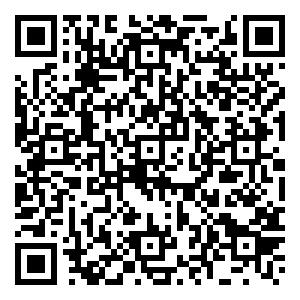
计量
- PDF下载量: 30
- 文章访问数: 1266
- 引证文献数: 0