基于改进Mask R-CNN模型的粘连烟丝识别方法
Adhesive tobacco shreds recognition method based on improved Mask R-CNN model
-
摘要: 为精准识别并高效分割粘连烟丝,提出一种基于改进掩码区域卷积神经网络模型(Mask R-CNN)的粘连烟丝识别方法。首先,采集粘连烟丝图像,通过图像增强操作将数据集扩充到训练模型所需的样本数量;其次,在Mask R-CNN模型的基础上对训练样本中的粘连烟丝图像进行边缘特征提取、分形特征提取,获得更清晰、连续的图像边缘特征信息和纹理特征信息;再次,将原始特征、边缘特征、分形特征进行融合以充分利用不同层次的特征信息,丰富底层特征;最后,通过引入混合注意力机制关注特征图的通道和空间维度,从而提高粘连烟丝识别的效率和准确性。模型性能对比结果表明:基于改进Mask R-CNN模型的识别方法的平均交并比(Avg.MIoU)为85.29%,类别平均像素准确率(Avg.MPA)为84.33%,其能够快速、准确地识别并分割出单根烟丝,识别效果优于Mask R-CNN和DeepLabV3+模型识别方法,可为后续烟丝宽度检测提供技术支持。
-
关键词:
- 粘连烟丝 /
- 改进Mask R-CNN模型 /
- 边缘特征提取 /
- 特征融合 /
- 混合注意力机制
Abstract: To achieve accurate identification and efficient segmentation of adhesive tobacco shreds, a method for adhesive tobacco shreds recognition based on an improved Mask R-CNN (Mask Region-based Convolutional Neural Network) model was proposed. Firstly, adhesive tobacco shreds images were collected, and the dataset was augmented through image enhancement operations to expand it to the required sample size for training the model. Secondly, edge feature extraction and fractal feature extraction were performed on the adhesive tobacco shreds images in the training set based on Mask R-CNN, resulting in clearer and more continuous image edge features and texture feature information. Subsequently, the original features, edge features, and fractal features were fused to fully utilize features at different levels and enrich low-level features. Finally, by introducing a hybrid attention mechanism that focused on both channel and spatial dimensions of feature maps, the efficiency and accuracy of tobacco shred recognition were improved. Experimental results showed that the mean intersectionover union (Avg.MIoU) of the recognition method based on the improved Mask R-CNN model was 85.29%, and the mean class pixel accuracy (Avg.MPA) was 84.33%, under different adhesion conditions enabling precise identification of tobacco shreds and outperforming the original Mask R-CNN and DeepLabV3+ models. This method could rapidly and accurately identify and segment adhesive tobacco shreds, providing technical support for subsequent tobacco shred width detection. -
-
[1]
孙东亮,张大波,李艳,等.烟丝长度及其混料配比对细支卷烟质量特性的影响[J].烟草科技,2019,52(11):87-95.
-
[2]
魏甲欣,李琪,马飞,等.基于图像识别的烟丝结构检测及烟丝组分分析[J].轻工学报,2022,37(3):82-87.
-
[3]
刘洪坤,邓国栋,李斌,等.基于变径圆的烟丝宽度图像检测方法[J].烟草科技,2022,55(2):77-83.
-
[4]
尹静.切丝质量故障的诊断分析[J].科技资讯,2020,18(7):53-54.
-
[5]
邓国栋,席年生,李洪涛,等.造纸法再造烟叶单独制丝关键工序加工条件对加工质量的影响[J].烟草科技,2014,47(3):9-13.
-
[6]
李金学,高尊华,杨帆,等.烟支内烟丝分布对卷烟质量的影响[J].烟草科技,2004,37(8):11-12
,15. -
[7]
赵佳成,高辉,王慧,等.切丝宽度对烟丝结构及其分布稳定性的影响[J].云南农业大学学报(自然科学),2017,32(4):668-677.
-
[8]
杨光露,鲁晓平,李琪,等.基于改进轻量化YOLOv5s的卷烟厂烟草粉螟视觉检测方法[J].轻工学报,2023,38(6):102-109.
-
[9]
周飞燕,金林鹏,董军.卷积神经网络研究综述[J].计算机学报,2017,40(6):1229-1251.
-
[10]
金保华,殷长魁,张卫正,等.基于机器视觉的苹果园果实识别研究综述[J].轻工学报,2019,34(2):71-81.
-
[11]
高震宇,王安,董浩,等.基于卷积神经网络的烟丝物质组成识别方法[J].烟草科技,2017,50(9):68-75.
-
[12]
钟宇,周明珠,徐燕,等.基于残差神经网络的烟丝类型识别方法的建立[J].烟草科技,2021,54(5):82-89.
-
[13]
牛群峰,袁强,靳毅,等.基于改进VGG16卷积神经网络的烟丝类型识别[J].国外电子测量技术,2022,41(9):149-154.
-
[14]
刘江鹏,牛群峰,靳毅,等.基于高效通道注意力机制与多尺度特征融合的烟丝图像识别方法研究[J].河南农业科学,2022,51(11):145-154.
-
[15]
中华人民共和国国家质量监督检验检疫总局,中国国家标准化管理委员会.烟草及烟草制品调节和测试的大气环境:GB/T 16447—2004[S].北京:中国标准出版社,2005.
-
[16]
HE K M,GKIOXARI G,DOLLÁR P,et al.Mask R-CNN[J].IEEE Transactions on Pattern Analysis and Machine Intelligence,2020,42(2):386-397.
-
[17]
WU J,WEI X Z.Omnidirectional gradient and its application in stylized edge extraction of infrared image[C]//2022 International Conference on Image Processing,Computer Vision and Machine Learning (ICICML).Xi'an:IEEE,2022:98-102.
-
[18]
ZHANG Y F,FAN Q L,BAO F X,et al.Single-image super-resolution based on rational fractal interpolation[J].IEEE Transactions on Image Processing:a Publication of the IEEE Signal Processing Society,2018,27(8):3782-3797.
-
[19]
张宸嘉,朱磊,俞璐.卷积神经网络中的注意力机制综述[J].计算机工程与应用,2021,57(20):64-72.
-
[20]
郭列,张团善,孙威振,等.融合空间注意力机制的图像语义描述算法[J].激光与光电子学进展,2021,58(12):329-338.
-
[1]
-
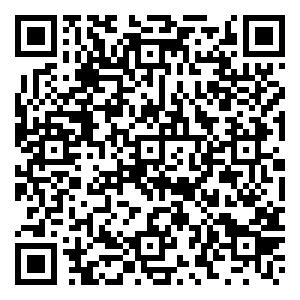
计量
- PDF下载量: 29
- 文章访问数: 1243
- 引证文献数: 0