基于兴趣度剪枝的Apriori优化算法
Optimized Apriori algorithm based on interestingness measure pruning
-
摘要: 鉴于关联规则挖掘中的Apriori算法在挖掘潜在有价值、低支持度模式时效率较低,因此提出一种优化的Apriori挖掘算法,即在频繁项集挖掘中引入项项正相关兴趣度量剪枝策略,有效过滤掉非正相关长模式和无效项集,从而扩大了可挖掘支持度阈值范围.实验结果表明,该算法是有效和可行的.Abstract: To solve the problem that the Apriori algorithm of mining association rules in database mining is not quite effective in the process of mining potentially valuable low-support patterns,an optimized apriori mining algorithm was proposed.This algorithm exploits an efficient pruning strategy which uses the interestingness measure to filter the non-positive correlated long model and invalid itemsets.The range of support threshold is expanded.The experimental results indicated that the given algorithm was efficient and feasible.
-
-
[1]
Jiawei Han,Micheline Kamber. Data Mining:Concepts and Techniques[M]. Second Edition. Beijing:China Machine Press,2006:147-172.
-
[2]
Agrawal R,Imielinski T,Swami A. Mining association rules between sets of items in large databases[C]//Proc of the ACM SIGMOD Conf on Mana of Data(SIGMOD'93), New York:ACM Press, 1993:207-216.
-
[3]
Omiecinski E. Ahemative interesting measures for mining associations[J]. IEEE Trans Knowledge and Data Eng, 2003,15:57.
-
[4]
李英杰.项约束频繁项集挖掘的新方法[J].计算机工程与应用,2009,45(3):161.
-
[5]
张玉芳,熊忠阳,彭燕,等.基于兴趣度含正负项目的关联规则挖掘方法[J].电子科技大学学报,2010,39(3):407.
-
[6]
王艳,刘双红,李玲玲.基于加权关联规则的选课推荐系统的构建[J].郑州轻工业学院学报:自然科学版, 2009,24(5):44.
-
[7]
Geng L Q,Hamilton H J. Interestingness measures for data mining:A survey[J]. ACM Comp Surveys, 2006, 38(3):9.
-
[8]
Brin S, Motwani R,Silverstein C. Beyond market baskets:generalizing association rules to correlations[C]//Proc ACM SIGMOD Int Conf on Mana of Data, Tucson:ACM Press, 1997:265-276.
-
[9]
Huang Wensue,Krneta Milorad,Lin Limin,et al. Associa tion bundle-A new pattern for association analysis[C]//Sixth IEEE Int Conf on Data Mining Workshops(ICDMW' 06) Washington:IEEE Computer Society,2006:601-605.
-
[1]
-
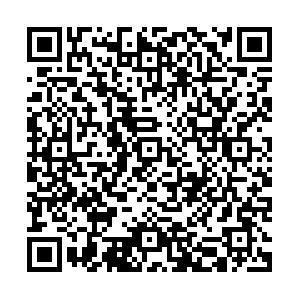
计量
- PDF下载量: 25
- 文章访问数: 749
- 引证文献数: 0