基于集成学习和基因本体标注库的细胞凋亡蛋白亚细胞位置预测
Predicting subcellular localization of apoptosis protein based on ensemble learning and Gene Ontology annotation database
-
摘要: 针对目前凋亡蛋白的亚细胞定位预测精度不高的问题,提出了基于集成学习和基因本体(GO)标注库的细胞凋亡蛋白亚细胞位置预测方法.该方法采用凋亡蛋白及其同源蛋白的GO特征,结合两层集成策略,预测凋亡蛋白的亚细胞位置.在第一层,依据不同同源蛋白个数生成多个特征向量集合,选取距离权重K近邻分类器作为个体分类器,训练多个子预测模型,并以多数投票的方式集成.在第二层,将第一层的集成模型作为子预测模型,以多数投票的方式集成不同近邻个数预测模型.Jackknife检验结果表明:该方法在CL317凋亡蛋白数据集上预测准确率达到96.2%,优于其他方法;此外,还有效降低了数据不均衡带来的影响.Abstract: In order to deal with the problem that the prediction accuracy of subcellular localization of apoptosis proteins is not high, a method of predicting subcellular localization of apoptosis protein based on ensemble learning and Gene Ontology (GO) annotation database was proposed. This method utilized the GO features of apoptosis proteins and their homologous proteins combined with the two layer integration strategy to predict subcellular localization of apoptosis proteins. In the first layer, several sets of feature vectors were formulated by the different number of homologous proteins, then it selected the distance weighted K-nearest neighbor classifier as individual classifier, trained sub-prediction models, and integrated these models by majority voting. In the second layer, the prediction model of the first layer was used as the sub-prediction model, and it integrated the different nearest neighbors' sub-prediction models by the majority voting. The results of Jackknife test showed that prediction accuracy of the method reaches 96.2% on the CL317 apoptosis proteins dataset, which was superior to other methods. In addition,this method could reduce the impact of the data imbalance.
-
-
[1]
EVAN G,LITTLEWOOD T.A matter of life and cell death[J].Science,1998,281(5381):1317.
-
[2]
REED J C,PATERNOSTRO G.Postmitochondrial regulation of apoptosis during heart failure[J].Proc Natl Acad Sci USA,1999,96(14):7614.
-
[3]
JACOBSON M D,WEIL M,RAFF M C.Programmed cell death in animal development[J].Cell,1997,88(3):347.
-
[4]
SCHULZ J B,WELLER M,MOSKOWITZ M A.Caspases as treatment targets in stroke and neurodegenerative diseases[J].Annals of Neurology,1999,45(4):421.
-
[5]
SUZUKI M,YOULE R J.Structure of Bax:Coregulation of dimer formation and intracellular localization[J].Cell,2000,103(4):645.
-
[6]
张松,黄波,夏学峰,等.蛋白质亚细胞定位的生物信息学研究[J].生物化学与生物物理进展,2007(6):573.
-
[7]
ZHOU G P,Doctor K.Subcellular location prediction of apoptosis proteins[J].Proteins:Structure,Function and Genetics,2003,50(1):44.
-
[8]
BULASHEVSKA A,EILS R.Predicting protein subcellular locations using hierarchical ensemble of Bayesian classifiers based on Markov chains[J].BMC Bioinformatics,2006,7(1):298.
-
[9]
ZHANG Z H,WANG Z H,ZHANG Z R,et al.A novel method for apoptosis protein subcellular localization prediction combining encoding based on grouped weight and support vector machine[J].FEBS Letters,2006,580(26):6169.
-
[10]
CHEN Y L,LI Q Z.Prediction of the subcellular location of apoptosis proteins[J].Journal of Theoretical Biology,2007,245(4):775.
-
[11]
CHEN Y L,ZHONG Q Z.Prediction of apoptosis protein subcellular location using improved hybrid approach and pseudo-amino acid composition[J].Journal of Theoretical Biology,2007,248(2):377.
-
[12]
DING Y,ZHANG T.Using Chou's pseudo amino acid composition to predict subcellular localization of apoptosis proteins:an approach with immune genetic algorithm-based ensemble classifier[J].Pattern Recognition Letters,2008,29(13):1887.
-
[13]
ZHANG L,LIAO B,LI D,et al.A novel representation for apoptosis protein subcellular localization prediction using support vector machine[J].Journal of Theoretical Biology,2009,259(2):361.
-
[14]
QIU J,LUO S,HUANG J,et al.Predicting subcellular location of apoptosis proteins based on wavelet transform and support vector machine[J].Amino Acids,2010,38(4):1201.
-
[15]
LIU T G,ZHENG X Q,WANG J,et al.Prediction of subcellular location of apoptosis proteins using pseudo amino acid composition:an approach from auto covariance transformation[J].Protein&Peptide Letters,2010,17(10):1263.
-
[16]
LIN H,WANG H,DING H,et al.Prediction of subcellular localization of apoptosis protein using Chou's pseudo amino acid composition[J].Acta Biotheoretica,2009,57(3):321.
-
[17]
GU Q,DING Y,JIANG X,et al.Prediction of subcellular location apoptosis proteins with ensemble classifier and feature selection[J].Amino Acids,2010,38(4):975.
-
[18]
YU X,ZHENG X,LIU T,et al.Predicting subcellular location of apoptosis proteins with pseudo amino acid composition:approach from amino acid substitution matrix and auto covariance transformation[J].Amino Acids,2012,42(5):1619.
-
[19]
SARAVANAN V,LAKSHMI P T V.APSLAP:an adaptive boosting technique for predicting subcellular localization of apoptosis protein[J].Acta Biotheoretica,2013,61(4):481.
-
[20]
LIU T,TAO P,LI X,et al.Prediction of subcellular location of apoptosis proteins combining tri-gram encoding based on PSSM and recursive feature elimination[J].Journal of Theoretical Biology,2015,366:8.
-
[21]
HARRIS M A,CLARK J,IRELAND A,et al.The Gene Ontology (GO) database and informatics resource[J].Nucleic Acids Research,2004,32(Database issue):D258.
-
[22]
CAMON E,MAGRANE M,BARRELL D,et al.The Gene Ontology Annotation (GOA) Database:sharing knowledge in Uniprot with Gene Ontology[J].Nucleic Acids Research,2004,32(Database issue):D262.
-
[23]
CAMON E,MAGRANE M,BARRELL D,et al.The Gene Ontology Annotation (GOA) Project:Implementation of GO in SWISS-PROT,TrEMBL,and InterPro[J].Genome Research,2003,13(4):662.
-
[1]
-
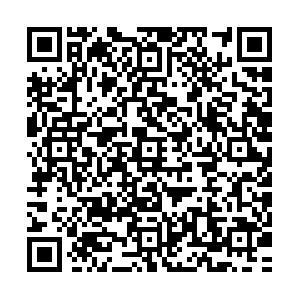
计量
- PDF下载量: 44
- 文章访问数: 913
- 引证文献数: 0