基于HCPS多层感知器的污水处理后氨氮浓度测量
Measurement of ammonia nitrogen concentration after sewage treatment based on HCPS multilayer perceptrons
-
摘要: 针对现污水处理后出水氨氮预测模型中隐含层神经元存在过大冗余而浪费资源的问题,提出了一种基于敏感度和互信息的混合增加删减的神经网络结构调整算法(HCPS).该算法重新定义了敏感度公式,利用敏感度和互信息自适应地调整网络结构,删除敏感度过低的隐含神经元,分裂过大的隐含层神经元,合并互信息过大的两个隐含层神经元.在污水处理基准仿真平台BSM1上的验证结果表明,HCPS算法可以获得更紧凑的网络结构,用于出水氨氮浓度预测精度较高.Abstract: In the existing prediction model of ammonia nitrogen in sewage treatment effluent, the hidden layer neurons excessively redundant, which wastes resources. A new structure adjustment algorithm (HCPS) based on sensitivity analysis (SA) and mutual information (MI) was proposed. The algorithm redefined the sensitivity formula, adaptively, adjusted the network structure by using sensitivity and mutual information, deleted hidden neurons with low sensitivity, divided hidden neurons with excessive sensitivity, and merged two hidden neurons with excessive mutual information. The results of verification on BSM1, a benchmark simulation platform for sewage treatment, showed that HCPS algorithm could obtain a more compact network structure, and the prediction accuracy of ammonia nitrogen concentration in effluent was higher.
-
-
[1]
DU R,PENG Y,CAO S,et al.Advanced nitrogen removal from wastewater by combining anammox with partial denitrification[J].Bioresource Technology,2015,179:497.
-
[2]
杨航涛,王双保,王成龙.基于分光光度法的水质氨氮检测系统设计[J].测控技术,2012,31(5):49.
-
[3]
乔俊飞,安茹,韩红桂.基于RBF神经网络的出水氨氮预测研究[J].控制工程,2016,23(9):1301.
-
[4]
ESFE M H,AFRAND M,WONGWISES S,et al.Applications of feedforward multilayer perceptron artificial neural networks and empirical correlation for prediction of thermal conductivity of Mg(OH)2-EG using experimental data[J].International Communications in Heat and Mass Transfer,2015,67(4):46.
-
[5]
ZHANG Y D,WANG S H,JI G L,et al.Fruit classification using computer vision and feedforward neural network[J].Journal of Food Engineering,2014,143(6):167.
-
[6]
FARAJZADEH J,FARD A F,LOTFI S.Modeling of monthly rainfall and runoff of Urmia lake basin using "feed-forward neural network" and "time series analysis" model[J].Water Resources and Industry,2014(7):38.
-
[7]
OONG T H,ISA N A.Adaptive evolutionary artificial neural networks for pattern classification[J].IEEE Trans Neural Netw,2011,22(11):1823.
-
[8]
WANG J H,WANG H Y,CHEN Y L,et al.A constructive algorithm for unsupervised learning with incremental neural network[J].Journal of Applied Research & Technology,2015,13(2):188.
-
[9]
PLATT J.A resource-allocating network for function interpolation[J].Neural Computation,1991,3(2):213.
-
[10]
LAURET P,FOCK E,MARA T A.A node pruning algorithm based on a Fourier amplitude sensitivity test method[J].IEEE Transactions on Neural Networks,2006,17(2):273.
-
[11]
ISLAM M M,SATTAR M A,AMIN M F,et al.A new adaptive merging and growing algorithm for designing artificial neural networks[J].IEEE Transactions on Systems Man and Cybernetics Part B,2009,39(3):705.
-
[12]
HAN H G,QIAO J F.A structure optimisation algorithm for feedforward neural network construction[J].Neurocomputing,2013,99:347.
-
[13]
GUO W,WEI H,ZHAO J,et al.Theoretical and numerical analysis of learning dynamics near singularity in multilayer perceptrons[J].Neurocomputing,2015,151:390.
-
[14]
HACINE-GHARBI A,RAVIER P,HARBA R,et al.Low bias histogram-based estimation of mutual information for feature selection[J].Pattern Recognition Letters,2012,33(10):1302.
-
[15]
GUO Y M,LIU Y G,ZENG G M,et al.An integrated treatment of domestic wastewater using sequencing batch biofilm reactor combined with vertical flow constructed wetland and its artificial neural network simulation study[J].Ecological Engineering,2014,64:18.
-
[16]
MIRBAGHERI B,EHTESHAMI M,BAGHERI Z.Modeling of a sequencing batch reactor treating municipal wastewater using multi-laye perceptron and radial basis function artificial neural networks[J].Process Safety and Environmental Protection,2015,93:111.
-
[17]
VIVIANO G,SALERNO F,CHIARA E,et al.Surrogate measures for providing high frequency estimates of total phosphorus concentrations in urban watersheds[J].Water Research,2014,64:265.
-
[18]
JEPPSSON U,PONS M N.The COST benchmark simulation model-current state and future perspective[J].Control Engineering Practice,2004,12(3):299.
-
[19]
HAN H G,QIAO J F.A structure optimization algorithm for feedforward neural network construction[J].Neurocomputing,2013,1(99):347.
-
[20]
CHEN C,YAN X.Optimization of a multilayer neural network by using minimal redundancy maximal relevance-partial mutual information clustering with least square regression[J].IEEE Transactions on Neural Networks and Learning Systems,2015,26(6):1177.
-
[1]
-
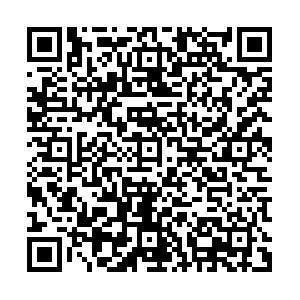
计量
- PDF下载量: 5
- 文章访问数: 1261
- 引证文献数: 0