ARL中Clean算法的并行化研究
Research on parallelization of Clean algorithm in ARL
-
摘要: 针对SKA算法参考库ARL中的去卷积算法运行效率低、无法满足海量数据实时处理的问题,提出了CPU和GPU协同工作模式下的并行化Clean算法.该方法将Clean算法中可以并行计算的步骤利用多线程在GPU上并行执行,将无法并行计算的步骤在CPU上串行执行.验证实验结果表明,在数据逐渐增大的过程中,并行化Clean算法比在CPU上的串行处理运行时间显著减少,当图达到4096像素×4096像素时,可以有10倍的提速.这说明并行化Clean算法在处理海量数据时,能够显著提高运算效率.Abstract: The deconvolution algorithm in the ARL of the SKA algorithm reference library is inefficient and cannot meet the needs of real-time processing of massive data. The parallelized Clean algorithm in the cooperative working mode of CPU and GPU was proposed. The steps of parallel computing in Clean algorithm were executed in parallel on GPU using multi-threads, and the steps in the Clean algorithm that couldn't be parallelized were executed serially on the CPU. The results showed that the running time of parallel Clean algorithm under CPU and GPU cooperative mode was significantly shorter than that under CPU. When the image size reached 4096×4096, the parallel Clean algorithm GPU cooperative mode could be speeded up by 10 times, which showed that the parallel Clean algorithm could significantly improve the efficiency of operation when dealing with massive data.
-
Key words:
- ARL /
- deconvolution algorithm /
- CUDA /
- parallel computing /
- Clean algorithm
-
-
[1]
DABBECH A,FERRARI C,MARY D,et al.Moresane:model reconstruction by synthesis-analysis estimators-a sparse deconvolution algorithm for radio interferometric imaging[J].Astronomy & Astrophysics,2015,576:7.
-
[2]
BROEKEMA P C,VAN NIEUWPOORT R V,BAL H E.The square kilometre array science data processor:Preliminary compute platform design[J].Journal of Instrumentation,2015,10(7):14.
-
[3]
VAN HEERDEN E,KARASTERGIOU A,ROBERTS S J,et al.New approaches for the real-time detection of binary pulsars with the Square Kilometre Array (SKA)[C]//General Assembly and Scientific Symposium.Piscataway:IEEE,2014:1-4.
-
[4]
LA CAMERA A,SCHREIBER L,DIOLAITI E,et al.A method for space-variant deblurring with application to adaptive optics imaging in astronomy[J].Astronomy & Astrophysics,2015,579:1.
-
[5]
HÖGBOM J A.Aperture synthesis with a non-regular distribution of interferometer baselines[J].Astronomy and Astrophysics Supplement Series,1974,15:417.
-
[6]
BHATNAGAR S,CORNWELL T J.Scale sensitive deconvolution of interferometric images-I:adaptive scale pixel (Asp) decomposition[J].Astronomy & Astrophysics,2004,426(2):747.
-
[7]
CORNWELL T J.Multiscale CLEAN deconvo-lution of radio synthesis images[J].IEEE Journal of Selected Topics in Signal Processing,2008,2(5):793.
-
[8]
RAU U,CORNWELL T J.A multi-scale multi-frequency deconvolution algorithm for synthesis imaging in radio interferometry[J].Astronomy & Astrophysics,2011,532:71.
-
[9]
ZHANG L,ZHANG M,LIU X.The adaptive-loop-gain adaptive-scale Clean deconvolution of radio interferometric images[J].Astrophysics & Space Science,2016,361(5):153.
-
[10]
CHENG J,XU L,LU Z,et al.Application of wavelet clean for Mingantu Spectral Radio-heliograph imaging[C]//2017 International Symposium on Intelligent Signal Processing and Communication Systems (ISPACS).Piscataway:IEEE,2017:81.
-
[11]
BOSE R.Lean Clean:deconvolution algorithm for radar imaging of contiguous targets[J].IEEE Transactions on Aerospace and Electronic Systems,2011,47(3):2190
-
[12]
CHEN L,LI L M,WAN G C,et al.A modified Clean algorithm for improving aperture synthesis observations of radio astronomy[C]//Progress in Electromagnetic Research Symposium.Piscataway:IEEE,2016:144.
-
[13]
HUANG X,WANG K,HUANG L,et al.GPU implementation for lo-regularized blind motion deblurring[C]//IEEE International Conference on Progress in Informatics and Computing.Piscataway:IEEE,2016:597-601.
-
[14]
SHERRY M,SHEARER A.IMPAIR:massively parallel deconvolution on the GPU[C]//Image Processing:Algorithms and Systems XI.[S.l.]:[s.n.],2013,8655(1):84-94.
-
[15]
SANDERS J,KANDROT E.CUDA by example[M].Boston:Addison-Wesley Professional,2010:18-25.
-
[16]
Nvidia.CUDA C best practices guide[M].American:Nvidia,2012.
-
[1]
-
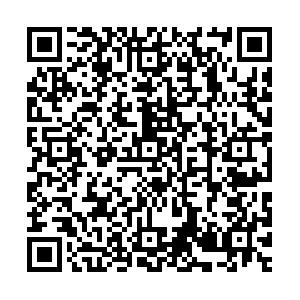
计量
- PDF下载量: 5
- 文章访问数: 1448
- 引证文献数: 0