语义核SVM结合改进EMD跨越语义鸿沟
Crossing semantic gap by semantic kernel SVM combined with improved EMD
-
摘要: 针对跨越语义鸿沟方法中未考虑文本间语义相关性和样本数量增加时计算量过大的问题,提出了一种语义核SVM结合改进EMD跨越语义鸿沟方法.该方法首先考虑到文本特征间的语义关系,提取与图像共生的文本关键词,结合HowNet通用本体库和内部统计特征构造语义核函数,然后将语义核函数嵌入SVM进行关键词分类,得到最佳候选关键词,从而解决文本间语义相关性问题;再通过最佳减小矩阵对EMD算法进行改进,从而减小计算量.对比实验结果表明,该方法充分利用了与图像共生的文本特征间的语义关系,标注准确率明显高于其他3种方法,且标注时间缩短为其他方法的1/5左右.Abstract: Aiming at the problem that the semantics relation among texts is not considered and the amount of computation is too large while samples increases in crossing semantic gap methods,a method of crossing semantic gap was proposed based on semantic kernel SVM combined with improved EMD. Firstly, to solve the semantic relation problem among texts, the method constructed the semantic kernel function based on taking semantic relations of text features into consideration, extracting text features coexisting with images and combining HowNet common ontology repository.Then the semantic kernel function was embedded into the SVM to classify keywords for obtaining best candidate words. Secondly, the algorithm improved EMD with best decreasing matrix to cut down the amount of computation. The experiment result showed that the method proposed takes full consideration of semantic relation in the texts related,the annotation accuracy rate was obviously higher than the other 3 methods and the annotation time was cut down to 1/5 of before.
-
Key words:
- semantic gap /
- semantic kernel /
- SVM /
- improved EMD
-
-
[1]
WEI S,WEI Y,ZHANG L,et al. Heterogeneous data alignment for cross-media computing[C]//International Conference on Internet Multimedia Computing and Service.New York:ACM,2015:84.
-
[2]
LU Z,PENG Y. Image annotation by semantic sparse recoding of visual content[C]//ACM International Conference on Multimedia. New York:ACM,2012:499.
-
[3]
许红涛,周向东,向宇,等.一种自适应的Web图像语义自动标注方法[J].软件学报,2010,21(9):2183.
-
[4]
VIKHAR P A,SHINKAR D V,MISHRA N. Improving the performance of CBIR system using relevance feedback[C]//International Conference and Workshop on Emerging Trends in Technology.New York:ACM,2010:554.
-
[5]
SMEULDERS A W M,WORRING M,SANTINI S,et al.Content-based image retrieval at the end of the early years[J].IEEE Transactions on Pattern Analysis & Machine Intelligence,2000,22(12):1349.
-
[6]
CAO Y,LONG M,WANG J,et al. Deep visual-semantic hashing for cross-modal retrieval[C]//Proceedings of the 22nd ACM SIGKDD International Comference.New York:ACM,2016:1445.
-
[7]
WANG B,YANG Y,XU X,et al.Adversarial cross-modal retrieval[C]//ACM on Multimedia Conference.New York:ACM,2017:154.
-
[8]
TSENG V S,SU J H,WANG B W,et al.Web image annotation by fusing visual features and textual information[C]//ACM Symposium on Applied Computing.New York:ACM,2007:1056.
-
[9]
ELAIDI H,BENABBOU Z,ABBAR H. A comparative study of algorithms constructing decision trees:ID3 and C4.5[C]//LOPAL'18 Proceedings of the International Conference on Learning and Optimization Algorithms:Theory and Applications. New York:ACM,2018:26.
-
[10]
MAI F,HUANG L,TAN J,et al.The research of semantic kernel in SVM for chinese text Classification[C]//2017 International Conference on inteligent Information Processing.New York:ACM,2017:1.
-
[11]
SIOLAS G. Support vector machines based on a semantic kernel for text categorization[C]//Proceedings of the IEEE-INNS-ENNS International Joint Conference on Neural Networks. IEEE Computer Society.Piscataway:IEEE,2000:5205.
-
[12]
张玉峰,王志芳.文本分类中的语义核函数研究[J]. 情报科学,2010(7):970.
-
[13]
WAN X J,PENG Y X.The earth mover's distance as a semantic measure for document similarity[C]//Proceedings of the 14th ACM International Conference on Information and Knowledge Management.New York:ACM,2005:301.
-
[14]
SHI J,MALIK J.Normalized cuts and image segmentation[J]. IEEE Transactions on Pattern Analysis and Maching Intelligence,2000,22(8):888.
-
[1]
-
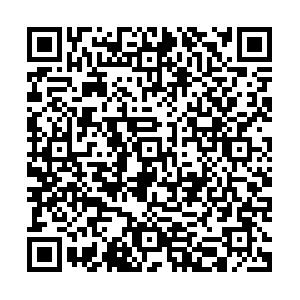
计量
- PDF下载量: 21
- 文章访问数: 1761
- 引证文献数: 0