青花瓷纹饰的艺术风格迁移研究
Research on the transfer of artistic style of blue-and-white porcelain decoration
-
摘要: 现代仿古、“互联网+”是高品质陶瓷的发展趋势,将陶瓷纹饰的艺术风格引入当代产品,实现时空融合及艺术再创造具有重要意义。以青花瓷纹饰为研究对象,将近现代景观画作为内容,叠加随机高斯白噪声得到初始的风格迁移图像,利用预训练的卷积神经网络提取内容图像和生成图像的内容特征,提取瓷器纹饰的风格图像和生成图像的风格特征,并分别计算内容损失和风格损失,通过计算损失函数并迭代至最小化,最终生成具有浓郁青花瓷韵味的风格迁移图像。实验结果表明,采用深度学习方法可以实现青花瓷纹饰和景观画作的融合,将学习到的青花瓷纹饰的特征成功迁移到景观画作上,不仅拓展了图像风格迁移的应用范围,而且产生了新的视觉体验,具有一定的艺术观赏性和创新性。Abstract: Modern antiques and Internet + were the development trends of high-quality ceramics. It was of great significance to introduce the artistic style of ceramic decoration into contemporary products and realize the fusion of time and space and artistic re-creation. Taking blue-and-white porcelain as the research object, the near-modern landscape painting was used as the content to superimpose random Gaussian white noise to obtain the initial style transfer image. The pre-trained convolutional neural network was used to extract the content image and the content feature of the generated image, and the style image of the porcelain decoration and the style feature of the generated image were extracted. The content loss and style loss were calculated separately. By the calculation of the loss function and iterating to minimize it, a style transfer image with a strong blue-and-white porcelain flavor was finally generated. The experimental results showed that the deep learning method could realize the integration of blue-andwhite porcelain decoration and landscape paintings, and successfully transfer the learned characteristics of blueand-white porcelain decoration to landscape paintings, which not only expanded the application scope of image style transfer, but also produced a new visual experience, with a certain artistic appreciation and innovation.
-
Key words:
- blue-and-white porcelain /
- decoration /
- painting /
- style transfer /
- convolutional neural network (CNN)
-
-
[1]
招伟培,罗宏,龙海仁,等.浅谈陕西铜川原材料及仿古、全抛釉瓷砖的坯体配方设计[J].佛山陶瓷,2020,30(7):31-36.
-
[2]
陈新,汪洲.现代仿古瓷的价值[J].景德镇陶瓷,2012,23(4):32.
-
[3]
赵国学.瓷话传统与现代[J].景德镇陶瓷,2019(6):12-13.
-
[4]
何笠农.传承与创新的当代陶瓷艺术[J].中国陶瓷,2012,48(2):62-65.
-
[5]
杜斐,陈明非,张云港.背景保持的服装片段风格迁移[J].软件导刊,2021,20(3):214-219.
-
[6]
邓筱,徐梦秋,吴铭,等.基于语义分割的织锦类自适应风格迁移算法[J].北京邮电大学学报,2021,44(1):117-123.
-
[7]
潘今一,王亚蒙,王伟,等.基于风格迁移和薄板样条的扩充汉字样本方法[J].浙江工业大学学报,2020,48(1):25-29
,39. -
[8]
李进,高静,陈俊杰,等.基于条件生成对抗网络的蒙古文字体风格迁移模型[J].中文信息学报,2020,34(4):58-62
,71. -
[9]
陈捷,徐戈.风格迁移算法在漆艺文创品设计中的运用[J].装饰,2020(3):82-85.
-
[10]
董荪,丁友东,钱昀.基于人工智能的风格迁移算法在动画特效设计中的应用[J].装饰,2018(1):104-107.
-
[11]
侯宇康,吕健,刘翔,等.基于神经风格迁移网络的民族图案创新方法[J].图学学报, 2020,41(4):606-613.
-
[12]
金思雨,覃京燕.基于计算机图像风格迁移的音乐可视化智能设计研究[J].包装工程,2020,41(16):193-198.
-
[13]
SIMONYAN K,ZISSERMAN A.Very deep convolutional networks for large-scale image recognition[C]//Internation Conference on Learning Representation 2015. America California:University of California Press,2015:1-14.
-
[14]
RUSSAKOVSKY O,DENG J,SU H, et al.ImageNet large scale visual recognition challenge[J].International Journal of Computer Vision,2015,115(3):211-252.
-
[15]
张月,刘彩云,熊杰.基于VGG-19图像风格迁移算法的设计与分析[J].信息技术与信息化,2020(1):70-72.
-
[16]
LI Y H,WANG N Y,LIU J Y,et al.Demystifying neural style transfer[C]//ACM.Proceedings of the 26th International Joint Conference on Artificial Intelligence.Australia Melbourne:AAAI Press,2017:2230-2236.
-
[17]
牟晋娟.基于深度学习的图像风格迁移技术的研究[J].电子元器件与信息技术,2019,22(4):86-89.
-
[1]
-
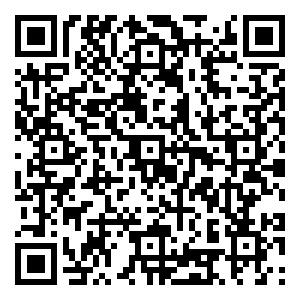
计量
- PDF下载量: 23
- 文章访问数: 2223
- 引证文献数: 0