SDN环境下不同机器学习算法的网络流量分类分析
Network traffic classification analysis of different machine learning algorithms in SDN environment
-
摘要: 为对比分析软件定义网络(SDN)环境下不同机器学习算法的网络流量分类效果,对Moore数据集进行了平衡处理,在机器学习平台RapidMiner上对K-近邻(KNN)、随机森林(RF)、支持向量机(SVM)和梯度提升决策树(GBDT)4种经典机器学习算法选取不同的分类特征进行分类实验.实验结果表明,较其他3种算法,GBDT算法可以在较短的时间内获得更好的分类效果.Abstract: In order to compare and analyze the network traffic classification effect of different machine learning algorithms in the software defined network (SDN) environment, the Moore dataset was balanced, and four classic machine learning algorithms including KNN, random forest (RF), support vector machine (SVM) and gradient lifting decision tree (GBDT) were supported on the machine learning platform RapidMiner to select different classification features for classification experiments. Experimental results showed that compared with the other three algorithms, the GBDT algorithm could obtain better classification results in a shorter time.
-
-
[1]
MCKEOWN N,ANDERSON T,BALAKRISHNAN H,et al.OpenFlow:Enabling innovation in campus networks[J].ACM SIGCOMM Computer Communication Review,2008,38(2):69.
-
[2]
吴艳.基于流量分类的智能SDN路由优化技术研究[D].杭州:浙江工商大学,2019.
-
[3]
胡孟婷.SDN网络流量态势评估及预测技术研究[D].成都:电子科技大学,2019.
-
[4]
刘佳美,徐巧枝.基于机器学习的SDN网络流量预测与部署策略[J/OL].计算机工程:1-7[2020-03-16
].https://doi.org/10.19678/j.issn.1000-3428.0056436. -
[5]
MOORE A W,PAPAGIANNAKI K.Toward the accurate identification of network applications[C]//Proceedings of International Workshop on Passive and Active Network Measurement.Heidelberg:Springer,2005:41.
-
[6]
彭芸,刘琼.Internet流分类方法的比较研究[J].计算机科学,2007(8):58.
-
[7]
SEN S,SPATSCHECK O,WANG D.Accurate,scalable in-network identification of p2p traffic using application signatures[C]//Proceedings of the Web Conference.Manhattan:[s.n.],2004:512.
-
[8]
NGUYEN T T T,ARMITAGE G.Training on multiple sub-flows to optimise the use of machine learning classifiers in real-world ip networks[C]//Proceedings of 2006 31st IEEE Conference on Local Computer Networks.Piscataway:IEEE,2006:369.
-
[9]
BERNAILLE L,TEIXEIRA R.Early recognition of encrypted applications[C]//Proceedings of International Conference on Passive and Active Network Measurement.Heidelberg:Springer,2007:165.
-
[10]
吴辉.基于模糊K-Means的网络流分类系统研究与实现[D].广州:广东工业大学,2016.
-
[11]
LI J,ZHANG S,LU Y,et al.Real-time P2P traffic identification[C]//Proceedings of 2008 IEEE Global Telecommunications Conference.Piscataway:IEEE,2008:1.
-
[12]
QUINLAN J R.C4.5:Programs for machine learning[M].Amsterdam:Elsevier,2014.
-
[13]
李平红,王勇,陶晓玲.支持向量机的半监督网络流量分类方法[J].计算机应用,2013,33(6):33.
-
[14]
何建涛.SDN中基于机器学习的DDoS攻击检测与防御方法研究[D].合肥:安徽大学,2019.
-
[15]
李兆斌,韩禹,魏占祯,等.SDN中基于机器学习的网络流量分类方法研究[J].计算机应用与软件,2019,36(5):75.
-
[16]
王赋翼.机器学习在流量分类中的应用[D].成都:电子科技大学,2019.
-
[17]
HAN H,WANG W Y,MAO B H.Borderline-SMOTE:A new over-sampling method in imbalanced data sets learning[C]//Proceedings of International Conference on Intelligent Computing.Heidelberg:Springer,2005:878.
-
[18]
张龙璨,柳斌,李芝棠.机器学习分类下网络流量的特征选取[J].广西大学学报(自然科学版),2011,36(S1):6.
-
[1]
-
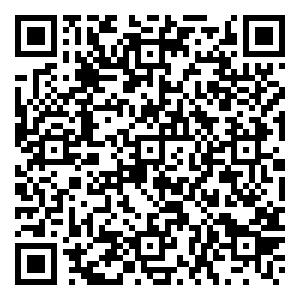
计量
- PDF下载量: 16
- 文章访问数: 2331
- 引证文献数: 0