一种基于深度学习的苦味肽精准预测方法
An accurate identification method of bitter peptides based on deep learning
-
摘要: 鉴于湿实验方法已无法满足快速鉴别苦味肽的需求,提出一种新颖的融合了传统手工特征和预训练深度特征的预测方法Bitter-Fus。该方法首先使用预训练蛋白质序列语言模型自动地从多肽序列中提取深度学习特征,然后将该特征输入长短期记忆(LSTM)网络中进行降维处理以保留与多肽序列最相关的深度特征,最后将降维后的深度特征与传统氨基酸组成(AAC)方法提取的手工特征融合并输入前馈神经网络中构建预测模型。验证实验结果表明:预测方法Bitter-Fus在10折交叉验证测试中获得了0.902的准确性和0.805的马修斯相关系数,在独立数据集测试中准确性和马修斯相关系数分别达到0.930和0.862,明显优于当前最先进的苦味肽预测方法BERT4Bitter和iBitter-SCM。Abstract: Given that wet experimental methods were no longer adequate for the rapid identification of bitter peptides, this paper presented Bitter-Fus, a novel predictive deep learning method incorporating traditional manual features and pre-trained deep features. Firstly, the method automatically extracted deep learning features from peptide sequences using a pre-trained protein sequence language model, then fed the deep learning features into a long short-term memory (LSTM) network for dimensionality reduction to retain the most relevant features. Finally, the reduced-dimensional deep features were fused with the manual features composed of traditional amino acids composition (AAC) method and passed into the feedforward neural network to construct a prediction model. The validation experimental results showed that the prediction method Bitter-Fus obtained an accuracy precision value of 0.902 and a Mathews correlation coefficient value of 0.805 in a 10-fold cross-validation, and an accuracy precision value of 0.930 and a Mathews correlation coefficient value of 0.862 in the independent dataset test, which significantly outperformed the current state-of-the-art bitter peptide prediction methods BERT4Bitter and iBitter-SCM.
-
Key words:
- bitter peptide /
- deep learning /
- feature extraction /
- feature fusion
-
-
[1]
刘桃妹.风味增强肽及其应用研究[J].江苏调味副食品,2014(3):5-8.
-
[2]
王知非,林璐,孙伟峰,等.苦味肽和苦味受体研究进展[J].中国调味品,2016,41(9):152-156.
-
[3]
毕继才,崔震昆,张令文,等.苦味传递机制与苦味肽研究进展[J].食品工业科技,2018,39(11):333-338.
-
[4]
司阔林,李志国,李玲玉,等.干酪苦味肽的形成及脱苦方法研究进展[J].食品工业,2021,42(3):267-271.
-
[5]
郭兴峰,魏芳,周祥山,等.苦味肽的形成机理及脱苦技术研究进展[J].食品研究与开发,2017,38(21):207-211.
-
[6]
应欣,张连慧,陈卫华.蛋白水解物苦味形成、评价及功能活性的研究进展[J].中国粮油学报,2017,32(12):141-146.
-
[7]
邓尚贵,余妙灵,甄兴华,等.苦味肽抗氧化活性延长食品保鲜[J].食品安全质量检测学报,2020,11(2):375-380.
-
[8]
杨保军,梁琪,宋雪梅.基于计算机虚拟技术研究牦牛乳硬质干酪苦味肽的抑菌活性差异[J].食品与生物技术学报,2021,40(12):75-87.
-
[9]
杨保军,梁琪,宋雪梅.牦牛乳干酪苦味肽ACE抑制活性表征的分子机制[J].中国食品学报,2022,22(5):8-17.
-
[10]
CHOU K C.Using amphiphilic pseudo amino acid composition to predict enzyme subfamily classes[J].Bioinformatics,2005,21(1):10-19.
-
[11]
BO W C,QIN D Y,ZHENG X,et al.Prediction of bitterant and sweetener using structure-taste relationship models based on an artificial neural network[J].Food Research International,2022,153:110974.
-
[12]
HUANG W K,SHEN Q C,SU X B,et al.BitterX:A tool for understanding bitter taste in humans[J].Scientific Reports,2016,6(1):23450.
-
[13]
ZHANG Y Q,ZHU G C,LI K W,et al.HLAB:Learning the BiLSTM features from the ProtBert-encoded proteins for the class I HLA-peptide binding prediction[J].Briefings in Bioinformatics,2022,23(5):bbac173.
-
[14]
WANG Z F,LEI X J. Prediction of RBP binding sites on circRNAs using an LSTM-based deep sequence learning architecture[J].Briefings in Bioinformatics,2021,22(6):bbab342.
-
[15]
GUO Y C,YAN K,LYU H W,et al.PreTP-EL:Prediction of therapeutic peptides based on ensemble learning[J].Briefings in Bioinformatics,2021,22(6):bbab358.
-
[16]
CHAROENKWAN P,YANA J,SCHADUANGRAT N,et al.iBitter-SCM:Identification and characterization of bitter peptides using a scoring card method with propensity scores of dipeptides[J].Genomics,2020,112(4):2813-2822.
-
[17]
CHAROENKWAN P,NANTASENAMAT C,HASAN M M,et al.BERT4Bitter:A bidirectional encoder representations from transformers (BERT)-based model for improving the prediction of bitter peptides[J].Bioinformatics,2021,37(17):2556-2562.
-
[18]
DEVLIN J,CHANG M W,LEE K,et al.BERT:Pre-training of deep bidirectional transformers for language understanding[C]//Association for Computational Linguistics.Proceedings of the 2019 Conference of the North American Chapter of the Association for Computational Linguistics:Human Language Technologies.Minneapolis,Minnesota:Association for Computational Linguistics,2019:4171-4186.
-
[19]
ELNAGGAR A,HEINZINGER M,DALLAGO C,et al.ProtTrans:Towards cracking the language of lifes code through self-supervised deep learning and high performance computing[J].IEEE Transactions on Pattern Analysis and Machine Intelligence,2022,44(10):7112-7127.
-
[20]
HOCHREITER S,SCHMIDHUBER J.Long short-term memory[J].Neural Computation,1997,9(8):1735-1780.
-
[21]
MINKIEWICZ P,DZIUBA J,IWANIAK A,et al.BIOPEP database and other programs for processing bioactive peptide sequences[J].Journal of AOAC International,2008,91(4):965-980.
-
[22]
GAUTAM A,CHAUDHARY K,KUMAR R, et al.In silico approaches for designing highly effective cell penetrating peptides[J].Journal of Translational Medicine,2013,11(1):74.
-
[23]
KUMAR R,CHAUDHARY K,CHAUHAN J S,et al.An in silico platform for predicting,screening and designing of antihypertensive peptides[J].Scientific Reports,2015,5(1):12512.
-
[1]
-
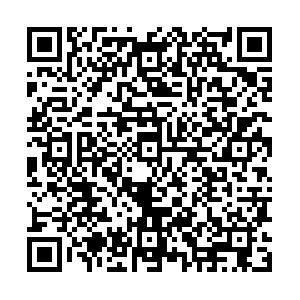
计量
- PDF下载量: 43
- 文章访问数: 3237
- 引证文献数: 0