CNN-RNN融合法在旋转机械故障诊断中的应用
Application of CNN-RNN fusion method in fault diagnosis of rotating machinery
-
摘要: 针对目前旋转机械故障诊断存在运算时间长、精度不高等问题,将CNN的特征提取能力和RNN时序处理能力相结合,提出了CNN-RNN融合分析法.该方法使用一维CNN网络提取特征数据,剔除受环境噪音等因素影响的无效信息且依然具有时序性,再由处理时序数据精度较高的RNN对该特征数据进行计算处理进而对旋转机械进行故障诊断.在测试集上的验证实验结果表明,该方法不需要手动提取特征数据,运算时间大约减少1/2,故障诊断精度提高约2%,具有可行性.Abstract: Aiming at the problems of current fault diagnosis of rotating machinery with long calculation time and low accuracy, a CNN-RNN fusion analysis method was proposed by combining the feature extraction capability of CNN and the processing capability of RNN timing. A one-dimensional CNN network was used to extract feature data, which removed invalid information affected by environmental noise and other factors and still had timeliness. Then, the RNN with high accuracy of processing time-series data calculated the feature data and then applied to the fault diagnosis of rotating machinery. The experimental results on the test set showed that the method did not require manual extraction of feature data, the computing time was reduced by about 1/2, and the accuracy of fault diagnosis was increased by about 2%.This method had feasibility.
-
-
[1]
陈长征,张省,虞和济.基于神经网络的旋转机械故障诊断研究[J].机械强度,2000,22(2):104.
-
[2]
任浩,屈剑锋,柴毅,等.深度学习在故障诊断领域中的研究现状与挑战[J].控制与决策,2017,32(8):1345.
-
[3]
YU H,HATZIVASSILOGLOU V.Towards answering opinion questions:Separating facts from opinions and identifying the polarity of opinion sentences[C]//Proceedings of Conference on Empirical Methods in Natural Language Processing.Sapporo:ACL,2003:129.
-
[4]
庄雨璇,李奇,杨冰如,等.基于LSTM的轴承故障诊断端到端方法[J].噪声与振动控制,2019,39(6):187.
-
[5]
张青青.基于改进AlexNet的滚动轴承变工况故障诊断研究[D].兰州:兰州理工大学,2019.
-
[6]
RAI A,UPADHYAY S H.A review on signal processing techniques utilized in the fault diagnosis of rolling element bearings[J].Tribology International,2016,96:289.
-
[7]
WANG L,WANG Z G,LIU S.An effectives multivariate time series classification approach using echo state network and adaptive differential evolution algorithm[J].Expert Systems with Applications,2016,43:237.
-
[8]
JOUIN M,GOURIVEAU R,HISSEL D,et al.Particle filter-based prognostics:Review,discussion and perspectives[J].Mechanical Systems and Signal Processing,2015,72/73:194.
-
[9]
赵光权,葛强强,刘小勇,等.基于DBN的故障特征提取及诊断方法研究[J].仪器仪表学报,2016,37(9):1946.
-
[10]
李巍华,单外平,曾雪琼.基于深度信念网络的轴承故障分类识别[J].振动工程学报,2016,29(2):340.
-
[11]
刘辉海,赵星宇,赵洪山,等.基于深度自编码网络模型的风电机组齿轮箱故障检测[J].电工技术学报,2017,32(17):156.
-
[12]
时培明,梁凯,赵娜,等.基于深度学习特征提取和粒子群支持向量机状态识别的齿轮智能故障诊断[J].中国机械工程,2017,28(9):1056.
-
[13]
李巍华,单外平,曾雪琼.基于深度信念网络的轴承故障分类识别[J].振动工程学报,2016,29(2):340.
-
[14]
侯文擎,叶鸣,李巍华.基于改进堆叠降噪自编码的滚动轴承故障分类[J].机械工程学报,2018,54(7):87.
-
[15]
HE K,ZHANG X,REN S,et al.Delving deep into rectifiers:Surpassing human-level performance on imagenet classification[C]//2015 IEEE International Conference on Computer Vision (ICCV).Piscataway:IEEE Conference Publications,2015:14.
-
[16]
张倩.基于共振解调原理和转速阶比谱分析的滚动轴承故障诊断方法研究[D].杭州:浙江大学,2012:37.
-
[17]
EL-THALJI I,JANTUNEN E.A summary of fault modelling and predictive health monitoring of rolling element bearings[J].Mechanical Systems and Signal Processing,2015,60/61:252.
-
[18]
VINYALS O,TOSHEV A,BENGIO S,et al.Show and tell:A neural image caption generator[C]//Proceedings of the 2015 IEEE Conference on Computer Vision and Pattern Recognition.Piscataway:IEEE Conference Publications,2015:47.
-
[19]
石鑫,朱永利.深度学习神经网络在电力变压器故障诊断中的应用[J].电力建设,2015,36(12):116.
-
[20]
吴国文,肖翱.基于深度学习神经网络的齿轮箱故障识别研究[J].网络安全技术与应用,2016(12):162.
-
[21]
侯荣涛,周子贤,赵晓平,等.基于堆叠稀疏自编码的滚动轴承故障诊断[J].轴承,2018(3):49.
-
[22]
张西宁,向宙,唐春华.一种深度卷积自编码网络及其在滚动轴承故障诊断中的应用[J].西安交通大学学报,2018,52(7):6.
-
[1]
-
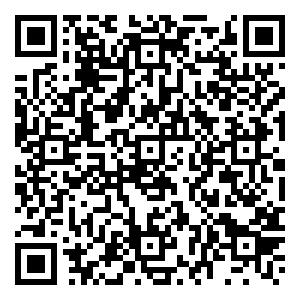
计量
- PDF下载量: 31
- 文章访问数: 2184
- 引证文献数: 0