虚拟筛选在化妆品功效原料发现中的应用进展
Application progress of virtual screening for the discovery of cosmetic efficacy ingredients
-
摘要: 基于虚拟筛选在化妆品功效原料发现的应用前景,对虚拟筛选的主要方法进行阐述,概述近年来虚拟筛选的发展趋势及其在化妆品功效原料发现中的应用现状。指出:虚拟筛选包含基于配体的虚拟筛选和基于受体的虚拟筛选两种方法,将不同虚拟筛选方法结合使用的混合法虚拟筛选在生物活性物发现中的应用不断增多;虚拟筛选应用于美白、祛痘、抗衰老等化妆品功效原料发现中已初见成效,然而,虚拟筛选本身正处于不断发展和完善的阶段,且利用虚拟筛选发现的某些先导化合物存在经皮吸收率低、未考虑功效物的外用特性等问题。未来可将化妆品功效原料的皮肤渗透性、安全风险评估等纳入到虚拟筛选的原则中,合理选择虚拟筛选方法,以促进虚拟筛选在化妆品功效原料研发中的实际应用。Abstract: Based on the application prospects of virtual screening for the discovery of cosmetic efficacy ingredients, the primary methods of virtual screening were reviewed, the development trends of virtual screening in recent years and its application cases in cosmetic efficacy ingredients were overviewed. It was concluded that virtual screening had ligand-based and receptor-based methods. The hybrid method combining diverse virtual screening was becoming increasingly used in bioactive compounds discovery, and it had achieved initial success in the functional ingredients, such as whitening, anti-acne, anti-aging, etc. However, virtual screening was still in the stage of continuous development and improvement, some lead compounds found by virtual screening had low transdermal absorption rate, and the external application characteristics of efficacy ingredients were not considered. In the future, the skin permeability and safety risk assessment of cosmetic efficacy ingredients should be incorporated into the principles of virtual screening, and the virtual screening methods should be selected reasonably, in order to promote the practical application of virtual screening in the development of cosmetics efficacy ingredients.
-
Key words:
- virtual screening /
- cosmetic /
- efficacy ingredient /
- pharmacophore
-
-
[1]
国家药品监督管理局.国家药监局关于发布《化妆品功效宣称评价规范》的公告(2021年第50号)[EB/OL].(2021-04-08
)[2022-08-28].https://www.nmpa.gov.cn/xxgk/ggtg/qtggtg/20210409160321110.html. -
[2]
王敏, 张晓娜, 徐鹤然, 等.《化妆品监督管理条例》对化妆品原料行业发展的影响[J].轻工学报, 2021, 36(5):92-101.
-
[3]
MACARRON R, BANKS M N, BOJANIC D, et al.Impact of high-throughput screening in biomedical research[J].Nature Reviews Drug Discovery, 2011, 10(3):188-195.
-
[4]
刘艾林, 杜冠华.虚拟筛选辅助新药发现的研究进展[J].药学学报, 2009, 44(6):566-570.
-
[5]
SOUSA S F, CERQUEIRA N M, FERNANDES P A, et al.Virtual screening in drug design and development[J].Combinations of Quantitative and High Throughput Screening, 2010, 13(5):442-453.
-
[6]
ACHARY P G R.Applications of quantitative structure-activity relationships (QSAR) based virtual screening in drug design:A review[J].Mini Reviews in Medicinal Chemistry, 2020, 20(14):1375-1388.
-
[7]
TROPSHA A, GOLBRAIKH A.Predictive QSAR modeling workflow, model applicability domains, and virtual screening[J].Current Pharmaceutical Design, 2007, 13(34):3494-3504.
-
[8]
SCIOR T, MEDINA F J L, DO Q T, et al.How to recognize and workaround pitfalls in QSAR studies:A critical review[J].Current Medicinal Chemistry, 2009, 16(32):4297-4313.
-
[9]
MURATOV E N, BAJORATH J, SHERIDAN R P, et al.QSAR without borders[J].Chemical Society Reviews, 2020, 49(11):3525-3564.
-
[10]
DRWAL M N, GRIFFITH R.Combination of ligand-and structure-based methods in virtual screening[J].Drug Discovery Today(Technologies), 2013, 10(3):e395-e401.
-
[11]
KUTLUSHINA A, KHAKIMOVA A, MADZHIDOV T, et al.Ligand-based pharmacophore modeling using novel 3D pharmacophore signatures[J].Molecules, 2018, 23(12):3094.
-
[12]
YAN X, LIAO C Z, LIU Z H, et al.Chemical structure similarity search for ligand-based virtual screening:Methods and computational resources[J].Current Drug Targets, 2016, 17(14):1580-1585.
-
[13]
CERETO-MASSAGUé A, OJEDA M J, VALLS C, et al.Molecular fingerprint similarity search in virtual screening[J].A Companion to Methods in Enzymology, 2015, 71:58-63.
-
[14]
HERNáNDEZ-SANTOYO A, TENORIO-BARAJAS A Y, ALTUZAR V, et al.Protein-protein and protein-ligand docking[M]//OGAWA T. Protein Engineering-Techno-logy and Application. Croatia:InTech, 2013:63-81.
-
[15]
ISSA N T, BADIAVAS E V, SCHüRER S.Research techniques made simple:Molecular docking in dermatology-a foray into in silico drug discovery[J].Journal of Investigative Dermatology, 2019, 139(12):2400-2408.
-
[16]
CERQUEIRA N M, GESTO D, OLIVEIRA E F, et al.Receptor-based virtual screening protocol for drug discovery[J].Arch Biochem Biophys, 2015, 582:56-67.
-
[17]
SETHI A, JOSHI K, SASIKALA K, et al.Molecular docking in modern drug discovery:Principles and recent applications[M]//GAITONDE V.Drug Discovery and Development-New Advances.London:IntechOpen, 2019:27-39.
-
[18]
SANDERS M P, MCGUIRE R, ROUMEN L, et al.From the protein's perspective:The benefits and challenges of protein structure-based pharmacophore modeling[J].MedChemComm, 2012, 3(1):28-38.
-
[19]
VÁZQUEZ J, LÓPEZ M, GIBERT E, et al.Merging ligand-based and structure-based methods in drug discovery:An overview of combined virtual screening approaches[J].Molecules, 2020, 25(20):4723.
-
[20]
ZHANG L, TAN J J, HAN D, et al.From machine learning to deep learning:Progress in machine intelligence for rational drug discovery[J].Drug Discovery Today, 2017, 22(11):1680-1685.
-
[21]
徐优俊, 裴剑锋.深度学习在化学信息学中的应用[J].大数据, 2017, 3(2):45-66.
-
[22]
GHASEMI F, MEHRIDEHNAVI A, PéREZ-GARRIDO A, et al.Neural network and deep-learning algorithms used in QSAR studies:merits and drawbacks[J].Drug Discovery Today, 2018, 23(10):1784-1790.
-
[23]
CRAMPON K, GIORKALLOS A, DELDOSSI M, et al.Machine-learning methods for ligand-protein molecular docking[J].Drug Discovery Today, 2022, 27(1):151-164.
-
[24]
DHASMANA A, RAZA S, JAHAN R, et al.Chapter 19 High-throughput virtual screening (htvs) of natural compounds and exploration of their biomolecular mechanisms:an in silico approach[M]//KHAN M S A, AHMAD I, CHATTOPADHYAY D. New Look to Phytomedicine.[S.L.]:Elsevier, 2019:523-548.
-
[25]
SELVARAJ C, CHANDRA I, SINGH S K.Artificial intelligence and machine learning approaches for drug design:challenges and opportunities for the pharmaceutical industries[J].Molecular Diversity, 2022, 26(3):1893-1913.
-
[26]
SABE V T, NTOMBELA T, JHAMBA L A, et al.Current trends in computer aided drug design and a highlight of drugs discovered via computational techniques:A review[J].Eur J Med Chem, 2021, 224:113705.
-
[27]
冯法晴, 刘有停, 董银卯.化妆品美白剂作用机制研究进展[J].香料香精化妆品, 2019(6):71-77.
-
[28]
AI N, WELSH W J, SANTHANAM U, et al.Novel virtual screening approach for the discovery of human tyrosinase inhibitors[J].Plos One, 2014, 9(11):e112788.
-
[29]
KIM B, LEE J Y, LEE H Y, et al.Hesperidin suppresses melanosome transport by blocking the interaction of Rab27A-melanophilin[J].Biomolecules & Therapeutics, 2013, 21(5):343-348.
-
[30]
JOUNG J Y, LEE H Y, PARK J, et al.Identification of novel rab27a/melanophilin blockers by pharmacophore-based virtual screening[J].Applied Biochemistry and Biotechnology, 2014, 172(4):1882-1897.
-
[31]
DESSINIOTI C, KATSAMBAS A D.The role of propionibacterium acnes in acne pathogenesis:facts and controversies[J].Clinics in Dermatology, 2010, 28(1):2-7.
-
[32]
GHOSH S, SINHA M, BHATTACHARYYA A, et al.A rationally designed multifunctional antibiotic for the treatment of drug-resistant acne[J].Journal of Investigative Dermatology, 2018, 138(6):1400-1408.
-
[33]
KIM J.Review of the innate immune response in acne vulgaris:activation of Toll-like receptor 2 in acne triggers inflammatory cytokine responses[J].Dermatology, 2005, 211(3):193-198.
-
[34]
GRABOWSKI M, MURGUEITIO M S, BERMUDEZ M, et al.Identification of a pyrogallol derivative as a potent and selective human TLR2 antagonist by structure-based virtual screening[J].Biochemical Pharmacology, 2018, 154:148-160.
-
[35]
JUGEAU S, TENAUD I, KNOL A, et al.Induction of toll-like receptors by Propionibacterium acnes[J].British Journal of Dermatology, 2005, 153(6):1105-1113.
-
[36]
TANG H C, HUANG H J, LEE C C, et al.Network pharmacology-based approach of novel traditional Chinese medicine formula for treatment of acute skin inflammation in silico[J].Computational Biology and Chemistry, 2017, 71:70-81.
-
[37]
BIRCH H L.Extracellular matrix and ageing[M]//ROBIN HARRIS J, I K V. Biochemistry and Cell Biology of Ageing:Part I Biomedical Science. Singapore:Springer, 2018:169-190.
-
[38]
KENNEDY K, CAL R, CASEY R, et al.The anti-ageing effects of a natural peptide discovered by artificial intelligence[J].International Journal of Cosmetic Science, 2020, 42(4):388-398.
-
[39]
LEPHART E D.Skin aging and oxidative stress:Equol's anti-aging effects via biochemical and molecular mechanisms[J].Ageing Research Reviews, 2016, 31:36-54.
-
[40]
SHEN Y, LIU C M, CHI K M, et al.Development of a machine learning-based predictor for identifying and discovering antioxidant peptides based on a new strategy[J].Food Control, 2022, 131:108439.
-
[41]
SUNG J Y, KIM S G, KIM J R, et al.SIRT1 suppresses cellular senescence and inflammatory cytokine release in human dermal fibroblasts by promoting the deacetylation of NF-κB and activating autophagy[J].Experimental Gerontology, 2021, 150:111394.
-
[42]
AZMINAH A, ERLINA L, RADJI M, et al.In silico and in vitro identification of candidate SIRT1 activators from Indonesian medicinal plants compounds database[J].Computational Biology and Chemistry, 2019, 83:107096.
-
[43]
TAO S, PARK S L, DE LA VEGA M R, et al.Systemic administration of the apocarotenoid bixin protects skin against solar UV-induced damage through activation of NRF2[J].Free Radical Biology and Medicine, 2015, 89:690-700.
-
[44]
LIEDER F, REISEN F, GEPPERT T, et al.Identification of UV-protective activators of nuclear factor erythroid-derived 2-related factor 2(Nrf2) by combining a chemical library screen with computer-based virtual screening[J].Journal of Biological Chemistry, 2012, 287(39):33001-33013.
-
[45]
YANG Y, WANG H P, WANG S Y, et al.GSK3β signaling is involved in ultraviolet B-induced activation of autophagy in epidermal cells[J].International Journal of Oncology, 2012, 41(5):1782-1788.
-
[46]
FU G, SIVAPRAKASAM P, DALE O R, et al.Pharmacophore modeling, ensemble docking, virtual screening, and biological evaluation on glycogen synthase kinase-3β[J].Molecular Informatics, 2014, 33(9):610-626.
-
[1]
-
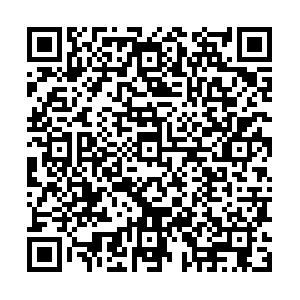
计量
- PDF下载量: 36
- 文章访问数: 3755
- 引证文献数: 0