基于过完备字典稀疏表示的云分类研究
Cloud classification research based on over complete dictionary sparse representation
-
摘要: 针对目前云类别自动识别方法较少的问题,提出了一种基于过完备字典稀疏表示的云分类的新方法.该方法用不同的云类型样本去建立自适应的过完备字典,提取字典特征,设计稀疏分类器,确定样本的云类型.仿真分析结果显示,本方法识别Ca,Cs&Cd,As&Ac,Ns&Cu,Cb云类型的准确率分别为100%,63.5%,90.3%,94.1%,98.2%,全局分类准确率为89.2%,优于支持向量机分类器和传统的稀疏表示分类器.Abstract: Aimed at the problem that automatic identification method for the cloud categories was less at present, a new method of cloud classification based on sparse representation of overcomplete dictionary was proposed. The method used different cloud types samples to establish an adaptive overcomplete dictionary, extracted dictionary features and designed sparse classifier to determine the type of cloud.The simulation analysis results showed that the classification accuracy of Ca,Cs&Cd,As&Ac,Ns&Cu,Cb were 100%, 63.5%, 90.3%, 94.1%, 98.2%, respectively.The overall classification accuracy was 89.2%. The classification accuracy was higher than the support vector machine classifier and the traditional sparse representation classifier.
-
Key words:
- satellite cloud /
- sparse representation /
- overcomplete dictionary
-
-
[1]
Dai D, Yang W.Satellite image classification via two-layer sparse coding with biased image representation[J].IEEE Geoscience and Remote Sensing Letters, 2011, 8(1):173.
-
[2]
Zhang L, Yang M, Feng X C.Sparse representation or collaborative representation:Which helps face recognition?[C]//Proceedings of 2011 IEEE International Conference on Computer Vision,Piscataway:IEEE,2011:471.
-
[3]
Naeger A R, Christopher S A, Ferrare R, et al.A new technique using infrared satellite measurements to improve the accuracy of the CALIPSO cloud-aerosol discrimination method[J].Geoscience and Remote Sensing,2013,51(1):642.
-
[4]
Han D, Yan W, Ren J Q,et al.Cloud type classification algorithm for CloudSatsatellite based on support vector machine[J].Atmospheric Science,2011(34):583.
-
[5]
周雪珺,杨晓非,姚行中.遥感图像的云分类和云检测技术研究[J].图学学报,2014,35(5):768.
-
[6]
金炜,符冉迪,范亚会,等.采用多模糊支持向量机决策融合的积雨云检测[J].光学精密工程,2014,22(12):3427.
-
[7]
Wright J, Yu L, Mairal J,et al.Sparse representation for computer vision and pattern recognition[J].Proceedings of the IEEE,2010,98(6):1031.
-
[8]
Sun X P, Wang J, She M,et al.Scale invariant texture classification via sparse representation[J].Neurocomputing,2013(122):338.
-
[9]
Sheng G F, Yang W, Yu L, et al.Cluster structured sparse representation for high resolution satellite image classification[C]//Proceedings of 2012 IEEE 11th International Conference on Signal Processing (ICSP),Piscataway:IEEE,2012:693.
-
[10]
Jaiswal N, Kishtawal C M.Automatic determination of center of tropical cyclone in satellite-generated IR images[J].Geoscience and Remote Sensing,2011,8(3):460.
-
[11]
尹雯,李元祥,周则明,等.基于稀疏表示的遥感图像融合方法[J].光学学报,2013,33(4):267.
-
[1]
-
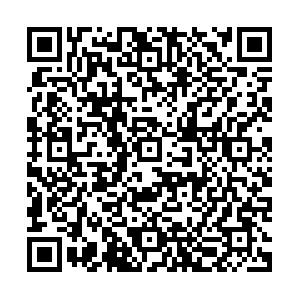
计量
- PDF下载量: 23
- 文章访问数: 1206
- 引证文献数: 0