基于稀疏编码的桥梁路面裂缝分类方法研究
Study on bridge floor crack classification method based on sparse coding
-
摘要: 针对桥梁安全和维护问题,提出了一种基于稀疏编码的桥梁路面裂缝分类方法.该方法从网上随机下载图片数据集作为训练集,减少人工标记的工作量,再用相机采集周围的桥梁路面裂缝图片作为测试集和验证集,针对这些高分辨率图像,采用改进的白化主成分分析进行降维,加速特征学习;针对裂缝图像特点,结合自学习算法,从大量未标识的数据集中提取尺度不变特征,经过改进的稀疏编码表示得到特征字典,并用空间金字塔进行池化;最后用线性支持向量机分类器进行分类.验证结果表明,与其他方法相比,本算法获得的分类准确率更高.Abstract: For the bridge safety and maintenance issues,a sparse coding method for the classification of bridge pavement cracks was proposed.Random downloading of image datasets from the Internet as a training set reduced the amount of manual tagging,and then the camera was used to capture the surrounding bridge crack images as test sets and validation sets.An improved whitening principal component analysis was used to reduce dimensions and accelerate feature learning for these high resolution images.A self-learning algorithm was used to extract scale-invariant features from a large number of unlabeled data sets based on the characteristics of crack images,and an improved sparse code representation was used to obtain a feature dictionary,and the space pyramid was used for pooling.Finally,multiclassification was performed using a linear support vector machine classifier. Experimental results showed that compared with other methods,the proposed algorithm could achieve higher classification accuracy.
-
-
[1]
ORTOLANO M,D'ELIA V,CALLEGARO L. A three-arm current comparator digitally assisted bridge for the comparison of arbitrary four terminal-pair impedances[J].IEEE Transactions on Instrumentation and Measurement,2017,66(6):1496.
-
[2]
CHEN Y,MEI T,WANG X J,et al.A bridge crack image detection and classification method based on climbing robot[C]//35th Chinese Control Conference.Piscataway:IEEE,2016:4037-4042.
-
[3]
陈瑶,梅涛,王晓杰,等.基于爬壁机器人的桥梁裂缝图像检测与分类方法[J].中国科学技术大学学报,2016,46(9):788.
-
[4]
韩洁,李盛阳,张涛.基于深度学习的遥感影像城市扩展方法研究[J].载人航天,2017,23(3):414.
-
[5]
石祥斌,房雪键,张德园,等.基于深度学习混合模型迁移学习的图像分类[J].系统仿真学报,2016,28(1):167.
-
[6]
徐佳,袁春琦,程圆娥,等.基于主动深度学习的极化SAR图像分类[J].国土资源遥感,2018,30(1):72.
-
[7]
夏梦,曹国,汪光亚,等.结合深度学习与条件随机场的遥感图像分类[J].中国图象图形学报,2017,22(9):1289.
-
[8]
BERRIEL R F,LOPES A T,DE SOUZA A F.Deep learning-based large-scale automatic satellite crosswalk classification[J].IEEE Geoscience and Remote Sensing Letters,2017,14(9):1513.
-
[9]
AFAN A,FAN Y Y. Automatic modulation classification using deep learning based on sparse autoencoders with nonegativity constraints[J].IEEE Signal Processing Letters,2017,24(11):1626.
-
[10]
O'SHEA T J,ROY T,CLANCY T C.Over-the-air deep learning based radio signal classification[J].IEEE Journal of Selected Topics Signal Processing,2018,12(1):168.
-
[11]
DE S,BRUZZONE L,BHATTACHARYA A,et al.A novel technique based on deep learning and a synthetic target database for classification of urban areas in PoISAR data[J].IEEE Journal of Selected Topics in Applied Earth Observations and Romote Sensing,2018,11(1):154.
-
[12]
SANTARA A,MANI K,HATWAR P,et al. BASS net:Band-adaptive spectral-spatial feature learning neural network for hyperspectral image classification[J].IEEE Transactions on Geoscience and Remote Sensing,2017,55(9):5293.
-
[13]
DEY D,CHATTERJEE B,DALAI S,et al. A deep learning framework using convolution neural network for classification of impulse fault patterns in transformers with increased accuracy[J].IEEE Transactions on Dielectrics and Electrical Insulation,2017,24(6):3894.
-
[14]
GUNDOGDU E,ALATAN A A. Good features to correlate for visual tracking[J].IEEE Transactions on Image Processing,2018,27(5):2526.
-
[15]
PEHLEVAN C,CHKLOVSHⅡ D B.Optimization theoty of Hebbian/anti-Hebbian networks for PCA and whitening[C]//Fifty-third Annual Allerton Conference on Communication,Control,and Computing (Allerton).Piscataway:IEEE,2015:1458-1465.
-
[16]
QUAN Y H,XU Y,SUN Y P,et al.Sparse coding for classification via discrimination ensemble[C]//2016 IEEE Conference on Computer Vision and Pattern Recognition.Piscataway:IEEE,2016:5839-5847.
-
[17]
CHUNDI P,SUBRAMANIAM M,SABET K,et al.Analyzing retinal optical coherence tomography images using differential spatial pyramid matching[C]//2016 IEEE 16th International Conference on Bioinformatics and Bioengineering. Piscataway:IEEE,2016:316-323.
-
[18]
URICAR M,TIMOFTE R,ROTHE R,et al.Structured output SVM prediction of apparent age,gender and smile from features[C]//2016 IEEE Conference on Computer Vision and Pattern Recognition Workshops. Piscataway:IEEE,2016:730-738.
-
[1]
-
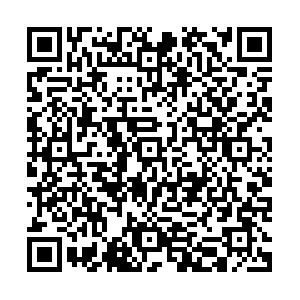
计量
- PDF下载量: 22
- 文章访问数: 1117
- 引证文献数: 0