基于AdaBoost多核支持向量机的跌倒检测研究
Research on fall detection based on Adaboost multiple kernel support vector machine
-
摘要: 针对传统的跌倒检测模型实时性低、误报率高的问题,提出AdaBoost多核支持向量机模型(ADB-MKSVM),用于对跌倒动作进行检测识别.该模型基于改进的AdaBoost模型框架,将多核支持向量机作为基分类器,并集合这些基分类器构成一个更强的最终分类器;依据人体动作数据分布和每次训练集中各样本的分类是否正确,以及上次的总体分类准确率,来确定每个样本的权值,采用权重动态分配的方法提高跌倒动作的识别率.测试结果表明,该模型具有良好的分类性能,且传感器绑于腰部位置可有效提高跌倒动作的检测效果,其准确率为99.33%,跌倒检出率为63.6%,跌倒检测错误率为1.62%.Abstract: Aim at the low real-time performance and high false alarm rate of the traditional fall detection model,AdaBoost multi-core support vector machine model (ADB-MKSVM) was proposed which was used to detect and identify the falling action. Based on the improved AdaBoost model framework, the model took multi-core support vector machine as the basis classifier and assembled these basis classifiers to form a stronger final classifier. According to the distribution of human movement data and whether the classification of each sample in each training set is correct or not, and the overall classification accuracy last time, the weight of each sample was determined. The dynamic weight allocation method was used to improve the recognition rate of the fall action. The test results showed that this model had good classification performance, and the method of binding the sensor on the waist position could effectively improve the detection effect of the fall action.The accuracy rate was 99.33%,the fall detection rate was 63.6%,and the false detection rate was 1.62%.
-
Key words:
- AdaBoost /
- multi-kernel learning /
- SVM /
- fall detection
-
-
[1]
MUBASHIR M,SHAO L,SEED L.A survey on fall detection:principles and approaches[J].Neurocomputing,2013,100:144.
-
[2]
王之琼,曲璐渲,隋雨彤,等.基于极限学习机的跌倒检测分类识别研究[J].中国生物医学工程学报,2014,31(4):418.
-
[3]
ZIGEL Y,LITVAK D,GANNOT I.A method for automatic fall detection of elderly people using floor vibrations and sound-Proof of concept on human mimicking doll falls[J].IEEE Transactions on Biomedical Engineering,2009,56(12):2858.
-
[4]
FOROUGHI H,ASKI B S,POURREZA H.Intelligent video surveillance for monitoring fall detection of elderly in home environments[C]//200811th Int Conf on Computer and Information Technology.Piscataway:IEEE,2008:219.
-
[5]
LEE J K,ROBINOVITCH S N,PARK E J.Inertial sensing-based pre-impact detection of falls involving near-fall scenarios[J].IEEE Transactions on Neural Systems and Rehabilitation Engineering,2015,23(2):258.
-
[6]
LAI C F,CHANG S Y,CHAO H C,et al.Detection of cognitive injured body region using multiple triaxial accelerometers for elderly falling[J].IEEE Sensors Journal,2011,11(3):763.
-
[7]
AZIZ O,ROBINOVITCH S N.An analysis of the accuracy of wearable sensors for classifying the causes of falls in humans[J].IEEE Transactions on neural Systems and Rehabilitation Engineering,2011,19(6):670.
-
[8]
CHENG W C,JHAN D M.Triaxial accelerometer-based fall detection method using a self-constructing cascade-AdaBoost-SVM classifier[J].IEEE Journal of Biomedical and Health Iinformatics,2013,17(2):411.
-
[9]
SHANY T,REDMOND S J,NARAYANAN M R,et al.Sensors-based wearable systems for monitoring of human movement and falls[J].IEEE Sensors Journal,2012,12(3):658.
-
[10]
KANGAS M,VIKMAN I,WIKLANDER J,et al.Sensitivity and specificity of fall detection in people aged 40 years and over[J].Gait & posture,2009,29(4):571.
-
[11]
TONG L,SONG Q,GE Y,et al.HMM-based human fall detection and prediction method using tri-axial accelerometer[J].IEEE Sensors Journal,2013,13(5):1849.
-
[12]
KANGAS M,KONTTILA A,WINBLAD I,et al.Determination of simple thresholds for accelerometry-based parameters for fall detection[C]//200729th Annual International Conference of the IEEE.Engineering in Medicine and Biology Society,Piscataway:IEEE,2007:1367.
-
[13]
GOH A T C.Back-propagation neural networks for modeling complex systems[J].Artificial Intelligence in Engineering,1995,9(3):143.
-
[14]
陈强,任雪梅.基于多核最小二乘支持向量机的永磁同步电机混沌建模及其实时在线预测[J].物理学报,2010,59(4):2310.
-
[15]
ZHANG Y,FU P,LIU W,et al.SVM classification for imbalanced data using conformal kernel transformation[C]//2014 International Joint Conference on Neural Networks (IJCNN).Piscataway:IEEE,2014:2894.
-
[16]
张凯军,梁循.一种改进的显性多核支持向量机[J].自动化学报,2014,40(10):2288.
-
[17]
亓晓振,王庆.一种基于稀疏编码的多核学习图像分类方法[J].电子学报,2012,40(4):773.
-
[18]
王新迎,韩敏.多元混沌时间序列的多核极端学习机建模预测[J].物理学报,2015,64(7):70504.
-
[19]
SUYKENS J A K,VANDEWALLE J.Least squares support vector machine classifiers[J].Neural Processing Letters,1999,9(3):293.
-
[20]
YANG X,TAN L,HE L.A robust least squares support vector machine for regression and classification with noise[J].Neurocomputing,2014,140:41.
-
[21]
SCHAPIRE R E.The boosting approach to machine learning:an overview[M]//Nonlinear estimation and classification.New York:Springer,2003:149.
-
[22]
WANG Z,JIANG M,HU Y,et al.An incremental learning method based on probabilistic neural networks and adjustable fuzzy clustering for human activity recognition by using wearable sensors[J].IEEE Transactions on Information Technology in Biomedicine,2012,16(4):691.
-
[23]
ALTUN K,BARSHAN B,TUNGEL O.Comparative study on classifying human activities with miniature inertial and magnetic sensors[J].Pattern Recognition,2010,43(10):3605.
-
[1]
-
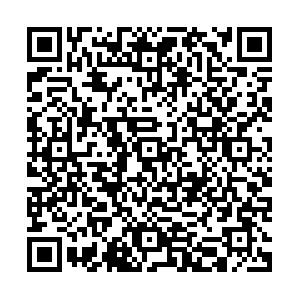
计量
- PDF下载量: 56
- 文章访问数: 2217
- 引证文献数: 0